fyvo
Learning is an act of tolerance and hope. The pursuit of learning as an adult is a complex commitment that brings about educational and emotional challenges across different settings and contexts. While assessment can foster metacognition and support learning, it can also create room for judgement and social pain. Thus, assessment models play a key role in setting the level of psychological safety and culture of a learning environment.
Today, millions of language learners -- especially those coming from developing countries -- feel demotivated and insecure when speaking a new language. Two reasons why this happens may be: lack of good pronunciation skills and lack of brain-friendly teaching and assessment models. In this project, I am going to explore a new way to assess and provide feedback to English language learners on a pronunciation training app, called FYVO (Find Your Voice).
Learning Vision
For this specific project, my goal is to propose a pronunciation training app for English learners in the United States. The real purpose behind this project, however, is not to help learners speak like Americans, but to help them become more confident -- not only to stay engaged in the app, but also to be able to use the language in their everyday activities and at the workplace.
I also realize that in order to build a real "brain-friendly" language learning experience and boost learner confidence, a larger focus on pronunciation training is not enough. A key component of language learning programs, which affects learners' motivation that cannot be overlooked is their feedback model -- especially for pronunciation exercises.
Learners and Other Stakeholders
Lack of good pronunciation skills may prevent students from engaging in English classes and with the English language as a whole. Just in Brazil, approximately 95% of the adult population cannot speak English. And out of the 5% that can, only 16% says they can speak the language at an advanced level (British Council Report, 2014). For context, public schools in Brazil offer English as a subject in high school. In 2021, I conducted 131 interviews with English learners in Brazil, and 64% have told me they had at least some level of insecurity to join conversations in English or even English classes. Out of the 64%, 83% mentioned their insecurity was connected to a lack of good pronunciation skills.
Boosting these English learners' confidence will help them advance in other areas of their language learning journeys. Difficulties in oral communication may stop students from continuing their language studies as well as make them feel self-conscious of their speaking. We also know that limited pronunciation skills can undermine learner’s self-confidence, restrict social interaction, and negatively influence estimations of a speaker’s credibility and abilities (Morley, 1998).
Today, most language programs do not focus on pronunciation training, although they do have pronunciation exercises. This happens for various reasons, from lack of teacher training (specifically in phonetics) to how difficult it may be to measure progress in pronunciation skills. After analyzing 20 of the most popular English language learning apps, two HGSE peers and I have concluded that most of them are mainly focused on grammar and vocabulary. Some newer apps are now specializing in pronunciation, focusing on the learner's confidence, but we feel that a brain-friendly learning experience also requires a good feedback and assessment model.
Our project for a pronunciation training app, FYVO, is focused initially on targeting foreigners in the United States. My team and I have so far interviewed international students and professionals from 8 different countries in order to investigate how pronunciation influences both their confidence to speak and their sense of fitting in their new English-speaking community -- and found 78% of them connect pronunciation to lack of confidence.
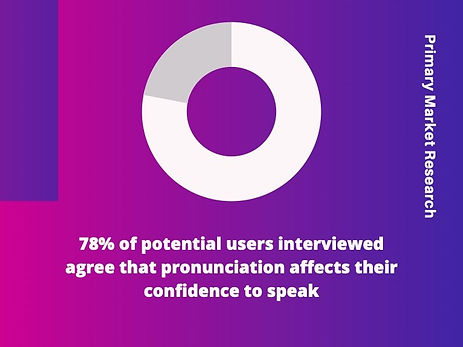
Curriculum, Technology, and Resources
FYVO is an app that is intended to act as your own personal pronunciation coach, based on TV content, leveraging speech recognition AI. In contrast to the popular language apps, it wouldn't just treat pronunciation as one component among a mix of lessons, but place it squarely in the center of the user experience. What truly distinguishes this idea, however, is the use of licensed media content as lesson material and a brain-friendly assessment model created exclusively for the app.
Here's a general idea of the initial journey a learner would go through on the app:
-
When a user signs up, the app collects key information to understand who they are (e.g. native language, level, context goals) and listens to them to then identify their unique pronunciation pattern.
-
Next, they choose who they want to speak like -- for example, Sean Connery from James Bond.
-
The app then compares the user's speech pattern to Sean's and generates a personalized set of easy and practical steps from intonation to phonetics so they can sound like Sean Connery.
-
Users can then practice quick exercises as they see precise activity metrics. Both the quick exercises and the metrics are intended to create deeper engagement and foster metacognition.
-
Users can also keep track of their progress by visualizing different metrics (e.g. activity on the app, intonation precision, pronunciation/phonetics troubleshooting), be challenged to improve specific areas of pronunciation, and be recommended personalized mini-lessons.
The app thereby harnesses both the emotional engagement of media and gamification, as well as of real-time data. This places it at a new intersection of language learning and entertainment. Most importantly, learners will be able to visualize their progress as they go through different phonetics exercises, so they know where they are in their unique language learning journeys.
Curriculum, Technology, and Resources
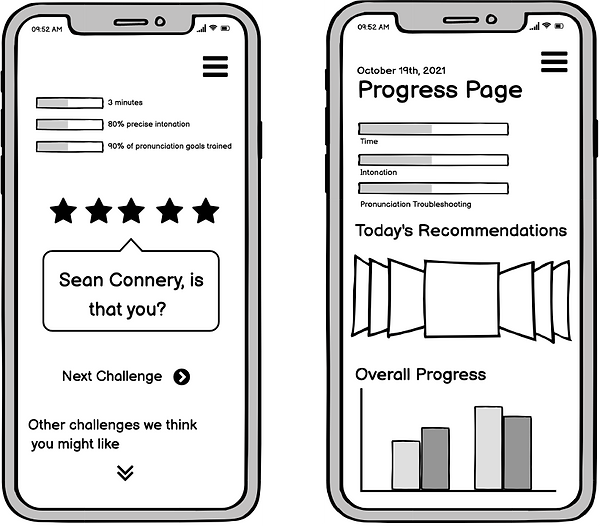
Real-time metrics have the power to engage, motivate, and reward learners as they navigate the app and complete "challenges". Here are two wireframes that show how a learner would see some of these metrics.
The wireframe on the left shows feedback at the end of an exercise. Here, we are looking into what types of feedback may keep learners engaged enough so they can move on to the next exercise. The "next challenge" button shows them another Sean Connery challenge, while the other arrows (pointing downwards) show different challenges they could try -- still aligned with their goals.
The wireframe of the right shows a "View Your Progress" page. In the first part of this wireframe, we can see three "easily measurable indicators" that we can use to give our users a sense of productivity and potentially surges of dopamine: time spend on the activities, intonation training, and pronunciation troubleshooting. Each individual has personal daily goals -- much like the Apple Watch's fitness activity feature. If, for example, your goal is to spend 30 minutes on the app a day, the bar will then indicate how much time, out of 30 minutes, you've already spent on the app. The constraint with the horizontal progress bar here is that if the user spends more than 30 minutes, we cannot represent it visually -- so for the real app, that bar will be changed to a circle or infinity symbol, so we can keep it going if users go beyond their personal goals.
The second part is a short section with recommended exercises based on their preferences/goals (using an algorithm to create a competency-based course). The third part would be their overall progress, which would show them a detailed report of which areas they have improved. Here, we can also include:
-
The users' biggest milestones (e.g. the video they spoke the fastest, or the day they spend most time on the app)
-
What specific phonemes they have improved more (or practiced more)
-
What the app recommends them to include as their goals -- e.g. "how about focusing on these phonics?" followed by phonemes that can then be assessed with the pronunciation troubleshooting bar
Strengths, Weaknesses, Threats, and Opportunities (SWOT)
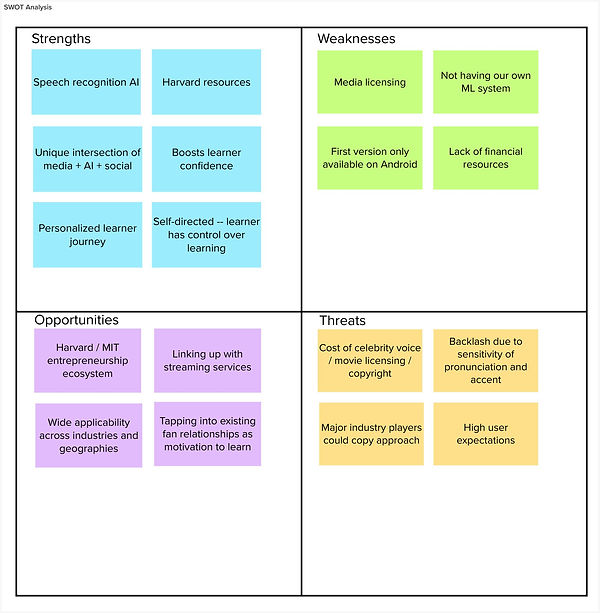
Scaling Up Technology-Enabled Improvements
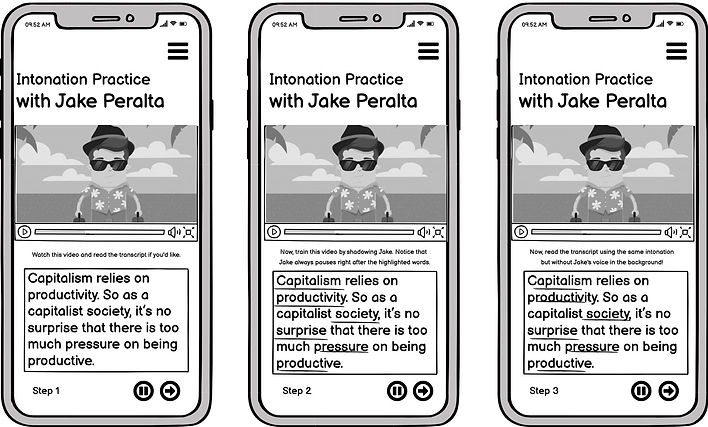
This is an example of an exercise that is specifically designed for intonation practice. Like all exercises, this one focuses on being a quick and effective type of activity -- where users can feel the reward and progress each time they practice. While they do the exercise, they are also progressing in the 3 activity metrics that are being constantly assessed when using the app (time, intonation training, and pronunciation troubleshooting). As more exercises like this one are designed, our ultimate goal remains to empower learners with confidence. In order to make sure that this is scalable and effective, we have elaborated our Primary Theory of Action and mapped out our constructs, along with some questions that will help us stay aligned with the actual demand for this app.
Theory of Action and Constructs
If non-native English speakers in the US reduce language barriers (when speaking and listening),
Then they can learn and practice English more confidently,
So that they find belonging in the US.
Here are some guidelines and maps for the creation of our learning experiences. Each exercise on the app is designed based on these principles.
Personalization and Brain-friendly Assessment for Online English Pronunciation Training
Language learning is non-linear. And because actual language learning involves forgetting and reminding oneself of new vocabulary, grammar rules, pronunciation, etc. multiple times and in different ways, language learning should be personalized if we want it to be more effective. While there is a smorgasbord of ways to make language learning more effective, this work focuses on how English speaking training can be personalized through AI on FYVO (Find Your Voice), an English speaking app started by students at HGSE.
Over the past decade, Artificial Intelligence has taken control over our daily interactions with apps. Apps have not only been learning our behaviors, but also changing or shaping them through personalization algorithms. In the language learning space, apps like Duolingo and Babble have used AI-driven approaches to help learners memorize vocabulary and structures as well as improve pronunciation skills (Holmes et al, 2019).
Although many apps have paved the way when it comes to using transformative technologies for language learning and pronunciation training, FYVO intends to put English speaking squarely at the center of the learning experience. As our goal in this project is to help learners improve speaking skills and give them agency to choose who they would like to speak like, FYVO will use Natural Language Processing, Machine Learning, and Artificial Intelligence. First, FYVO collects a speech sample from a learner and uses NLP for speech recognition. Second, the learner chooses a favorite TV star or public figure they would like to speak like. Third, FYVO uses ML to compare a speech sample of that learner with a speech sample of their chosen TV star (that involves breaking pronunciation down into phonemes, phonation, intonation, and speech flow). Finally, the AI-based app creates a personalized set of exercises for the learner to start with, and lets them choose what they would like to work on first in order to achieve the target pronunciation.
FYVO's approach has the potential to change how English speaking training apps help learners build confidence. The app would offer learners a library of quick lessons with personalized recommendations based on their pronunciation goals and preferred TV stars and genres. Real-time metrics are also shown to users as they go through exercises, helping them stay engaged and feeling proud of themselves. The overall experience of being able to choose who one would like to speak like and get instantaneous feedback related to effort is more similar to the real experience of learning a language confidently. In other words, instead of sequence of units and courses, FYVO aims to offer a language learning experience that is brain-friendly, non-linear, immersive, and fun.
FYVO, Personalized Learning, and A.I.
This app is intended to be a confidence-building learning experience. While most apps tell learners whether they are right or wrong, we believe that there is no “wrong” way to say or pronounce words. In order to make FYVO a way to create meaningful learning experiences to users, artificial intelligence will be used to leverage powerful approaches to learning that foster confidence. The main concept explored in this project is Self- regulated Learning, which would give us space to generate Personalized Learning and Effort-based Feedback.
Self-regulated learning happens when a learner controls their own learning process, and this control includes observing, mastering, and regulating (Garcia and Pintrich 1994). Self-Determination Theory proposes that human beings have three fundamental psychological needs: autonomy, competence, and relatedness (Deci and Ryan 2011; Ryan and Deci 2000). Autonomy refers to the sentiment of choice and concurrence with one’s actions; competence refers to the sentiment of being effective and capable; and relatedness refers to the sentiment of connecting to other individuals and groups (Ryan 2019).
Based on these theories, FYVO uses each exercise/feature as an opportunity to retrieve data from the user's speech and to not only suggest a highly personalized learning journey, but also to make sure learners feel self-efficacy. After each exercise, FYVO shows users real-time pronunciation metrics -- intonation precision, percentage of target phonemes trained, and percentage of speech fluidity -- and suggests followup exercises so users can choose to train the parts of speech that will make them feel more confident (according to their own target pronunciation and daily goals). But to make even more space for self- regulated learning and metacognition, the app allows users to choose which followup exercises they would like to do or skip -- giving them the agency to speak just the way they want.
To motivate learners, FYVO therefore measures effort, and instead of progressing down a long line of predetermined lessons, our learners receive real-time suggestions for new exercises at every step. With this, learners not only get the motivating feeling of moving forward, but also enjoy maximum agency, so that from the moment a learner opens the app, no two pathways are the same.
Finally, English is just as diverse as the people who speak it. Therefore, AI is the key in making sure that this language app will not do what every other language app has done so far: standardize a mid-western American accent or British RP. By learning the needs and preferences of each user, AI can not only create recommendation algorithms to exercises that fit the learner's needs and preferences, but also craft brain-friendly learning journeys.
What does it look like?
On other apps we have used daily -- such as Netflix, Spotify, and Apple Music -- we have the agency to navigate freely. That means we have the ability to choose songs or movies using a search bar and that navigation is in the format of a library. AI is used in these apps to then create personalized experiences for users, recommending playlists or shows according to each person's preferences and tastes. On the other side, language learning apps and online courses usually follow a more structured approach, with units and lessons. Recently, however, Quizlet launched its Learning Assistant in order to create personalized learning experiences -- which shows us a new trend in online learning.
It is my opinion that language learning is a non-linear process, which only works if the learner takes ownership of it. That means the learner is better able to feel self-efficacy and actually learn a language if they are able to find meaning in learning it. Sometimes "meaning" comes in different ways -- it may be a need or just a desire the learner has. On FYVO, "meaning" is the combination of being able to choose lines and artists from their favorite shows, movies, and interviews to train speaking and visualizing one's own progress taking shape.
Apple's fitness app works with the Apple Watch to retrieve and show users real-time metrics in order to keep them physically active and motivated to move. On FYVO, we would like to do something similar to change what assessment looks like. Ultimately, the effort-based assessment model is meant to boost confidence and motivation.
Here are some UI screens of FYVO:
Implementation and Limitations
"In order for AI to do its job, models need to be trained on data. However, data brings quite a few obstacles to the table" (Bayern, 2018). Because we are designing an English speaking training app -- and because of the diversity of users and accents -- huge amounts of data will be necessary to create a safe learning space for different learners. As aforementioned, the app is intended to help learners build confidence by finding their own voices. The biggest challenge in implementing this technology, however, is teaching different accents to the models being used.
Actors learn different accents from vocal coaches. Conversely, FYVO's models will learn different accents from data. The more the app is used, the more data we can get from different learners. So how can we make sure that learners are actually being assessed correctly? Again, our assessment consists of two parts: effort-based assessment with real-time metrics and recommended and optional exercises based on the user's performance and target pronunciation. So how will these recommendations be accurate? And would the app be biased if it recommends learners train a part of their speech they think is already perfect?
Other language learning apps have also been looking for ways to train their AI. As an example, ELSA Speak collected speech samples from thousands of learners around the world after going viral. Their goal, however, was to train their AI to learn what a neutral American accent sounds like compared to other non-native English speakers. Our goal is to make a learning experience where learners are not "corrected" because of their accent. That means we would have to collect data from speakers with different accents until the AI learns what learners really need in order to learn their target pronunciations.
As it is a hard task to quickly gather all these data and implement the app as we would like to, the app will need to be developed in different stages. First, market research will tell us what accents are more popularly demanded. Next, we will set the order in which FYVO will implement better accuracy to assess different pronunciation patterns. Nevertheless, in order to make sure any initial bias from the AI will not interfere in a learner's comfort to use the app, the app will start with fewer choices of accents and will use the metrics and self-regulated learning tools mentioned earlier in this work to make sure learners feel progress without being judged.
Works Cited
Bayern, Macy. "The 3 most overlooked limitations of AI in business." TechRepublic, 03 October 2018 www.techrepublic.com/article/the-3-most-overlooked-limitations-of-ai-in-business. Accessed 14 December 2021.
Deci, E. L., & Ryan, R. M. (2000). The “what” and “why” of goal pursuits: human needs and the self-determination of behavior. Psychological Inquiry, 11(4), 227–268.
Deci, E. L., & Ryan, R. M. (2011). Levels of analysis, regnant causes of behavior and wellbeing: the role of psychological needs. Psychological Inquiry, 22(1), 17–22. https://doi-org.ezp-prod1.hul.harvard.edu/10.1080/1047840x.2011.545978.
Garcia, T., & Pintrich, P. R. (1994). Regulating motivation and cognition in the classroom: The role self-schemas and self-regulatory strategies. In D. H. Schunk & B. J. Zimmerman (Eds.), Self-regulation of learning and performance: issues and educational applications (pp. 127–153). Hillsdale: Lawrence Erlbaum.
Holmes, W., Bialik, M., & Fadel, C. (2019). Artificial Intelligence in Education: Promises and Implications for Teaching and learning. (p. 140)
Luo, Y., Lin, J. & Yang, Y. Students’ motivation and continued intention with online self-regulated learning: A self-determination theory perspective. Z Erziehungswiss (2021).
Morley, J. (1998). Trippingly on the tongue: Putting serious speech/pronunciation instruction back in the TESOL equation. ESL Magazine, January/February, 20-23.
Ryan, R. M., & Deci, E. L. (2019). Chapter four—brick by brick: the origins, development, and future of self-determination theory. In A. J. Elliot (Ed.), Advances in motivation science (Vol. 6, pp. 111–156). Amsterdam: Elsevier.